Program of the courses
1. 10 July 2018: Perturbation of eigenvalues of matrices
2. 12 July 2018: Sylvester equation and perturbation of eigenvectors
3. 17 July 2018: Geometric mean of PSD matrices and Riemannian geometry
4. 19 July 2018: Bures-Wasserstein metric on PSD matrices
Contact: Marco Congedo, DIS, GIPSA-lab
Context of the Courses:
Several areas in physics and engineering, both traditional (like the theory of vibrations, elasticity, quantum mechanics, electrical networks) and more recent (like radar data, image processing, machine learning, brain-computer interfaces) draw upon tools from matrix analysis. This is also a rich and elegant mathematical theory in itself with connections to functional and harmonic analysis, differential geometry, and algebra, Multidimensional data often leads to correlation matrices. These, in turn, are positive definite. So, the theory of positive definite matrices has become very important in recent years.
Many problems in these applications require a notion of smoothing of data, or an averaging, which respects some structures, and has both good mathematical properties and is useful for computations. One such notion is that of the geometric mean. In the 1970's physicists and electrical engineers, followed by mathematicians, developed this for the case of two positive definite matrices. This became an effective tool for proving matrix inequalities arising in quantum statistical mechanics and in electrical networks. A good and useful definition for more than two matrices had been elusive and was found only in 2004. This involves ideas from Riemannian geometry and functional analysis. Here there have been very interesting developments. On the one hand the geometric mean has found major applications in the areas mentioned above. On the other, questions arising from the study of the mean have led to new understanding of some problems in geometry. A new subject called "matrix information geometry" has emerged.
Closely related to this is another area: the Bures-Wasserstein distance used in statistics and the theory of optimal transport. Here too there are connections with differential geometry, matrix inequalities, and computations.
This course of lectures will start with the basic tools of matrix analysis, and progress to a full discussion of the geometrical ideas needed for the theory. Prof. Rajendra Bhatia (Ashoka University, India)
Awards and Honours
- Hans Schneider Prize in Linear Algebra, 2016
- J. C. Bose National Fellow.
- Fellow, Third World Academy of Sciences.
- Fellow, Indian Academy of Sciences.
Notable Books
- Matrix Analysis, Graduate Texts in Mathematics, Springer-Verlag, New
York, 1996; Indian edition, 2000; Chinese edition 2010.
- Fourier Series, Mathematical Association of America, 2005.
- Positive Definite Matrices, Princeton University Press, 2007.
- Perturbation Bounds for Matrix Eigenvalues, SIAM Classics in Applied Mathematics, Philadelphia, 2007.
Fields of Interests
Analysis of matrices and linear operators: perturbation of eigenvalues and eigenvectors, matrix inequalities, operator functions, norm ideals of operators, connections with Fourier analysis, differential geometry, approximation problems, applications to numerical analysis, computations and mathematical physics.
Location : Chartreuse Room (Building D), GIPSA-lab Department Images and Signal, 11 rue des Mathématiques, Grenoble Campus, Saint Martin d'Hères
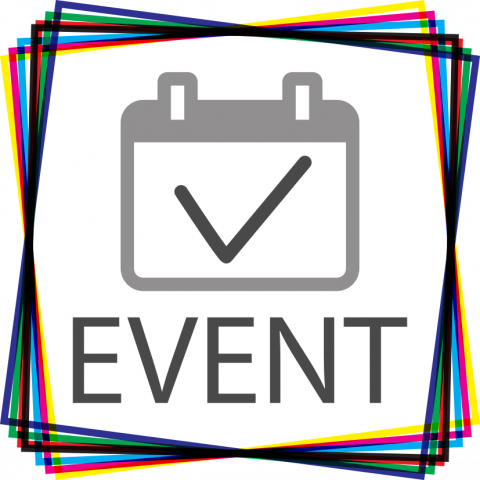