In a context where modeling, estimation, analysis and control theory for dynamical systems have reached a certain maturity for well-defined models and where uncertainties on the data, the operational environments, and the models themselves strongly gained relevance, MODUS team puts these issues at the heart of its research: uncertainties must be considered in the framework of guaranteed methodological approaches, to deal with problems strongly linked to the real world.
These issues lead to consider control and decision-making strategies that aim at going beyond classical closed-loop methods, by resorting also to the potentialities of modern optimization tools. Furthermore, the uncertainties related to missing measurements or partially unknown model parameters naturally motivate the interest on the fundamental problems of estimation, as for inverse problems, identification and observation. The developed methods will therefore combine classical tools based on Lyapunov or optimal control (calculus of variations, dynamic programming, MPC) for guaranteeing stability or optimality, with stochastic, set-based or learning-based techniques for dealing with uncertainties and large data sets.
In line with the projects already launched, and with the desire to further develop relationships with the socio-economic context, the application domains fall within the Energy/Mechatronics, Processes/Environment, Computing/Data and Health/Living contexts.
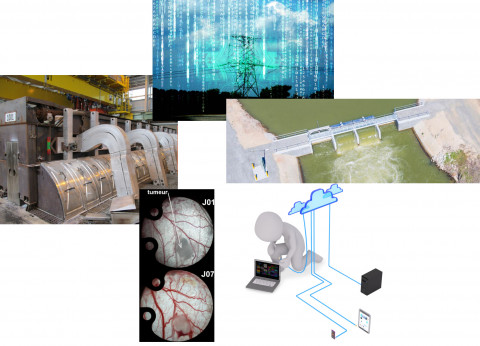